I’m a huge fan of using analytics to measure marketing. Rather than going with your gut, turn your customer data into marketing insights. One of the most popular concepts in marketing analytics is called lift. However, lift has some drawbacks, which another measure, called effect size, does not. Thus, it’s important to understand how to calculate lift and effect size.
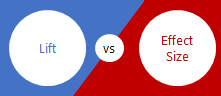
Understanding lift vs effect size
One of the most important topics in advanced analytics is how to measure the effect of a controlled experiment. In domains like sales and marketing, a firm that can execute and learn from experiments can create a sustainable competitive advantage.
What is lift?
In marketing, lift is the percent improvement of a target metric.
For example, suppose our advertising campaign averages a 2% clickthrough rate. Now, suppose that we modify our campaign. If our clickthrough rate improves from 2% to 2.5%, then we have a lift of 0.25 or 25%.
Drawbacks of lift
Lift is an attractive metric, because it is easy to understand, easy to measure, and easy to explain. A higher lift means better results.
Now, from a mathematical standpoint, the main drawback of lift is that it does not take randomness into account. We need to ask the question:
How much of our improvement was due to our actions, and how much was due to randomness?
Consider our previous example. If I told you, of the 25% lift, that 20% was due to randomness and only 5% was due to our actions, then our results sound less impressive.
The true improvement of our clickthrough rate was only from 2% to 2.1%, rather than to 2.5%. In this case, our lift was due more to luck than to good marketing design.
Using effect size to control for randomness
To control for randomness, we measure the effect size of our test. This allows us to quantify the statistical strength of our results.
Now, there are several ways to calculate effect size. When working with controlled experiments, one of the most popular is Cohen’s d.
Whereas lift only considers averages (e.g., average clickthrough rate), calculating Cohen’s d involves calculating standard deviation as well.
Calculate lift and effect size
So let’s do some math. First, let’s start off with some definitions. Let:
= Size of the first (or control) group
= Size of the second (or test) group
= Average of the first (or control) group
= Average of the second (or test) group
= Sample standard deviation of the first (or control) group
= Sample standard deviation of the second (or test) group
The formula for lift is:
The formula for Cohen’s d is:
where:
While the formula for Cohen’s d seems intimidating at first, it’s important to realize that it is quite straightforward. After we calculate our descriptive statistics, it is simple to plug values into the formula.
More important, of course, is understanding what these values mean.
Example: calculate lift and effect size
So let’s consider an example. Suppose that we operate an eCommerce store. We run a marketing campaign, as an A/B test, offering a promotion to incentivize customers to increase their order size. Hence, we want to track the effect size of the promotion on customer spend.
The raw data: control group vs test group
During the test, we captured 50 orders in the control (the A group) and 60 orders in the test group (the B group). Thus, our data looks like this.
Control | Test |
---|---|
$57 | $30 |
$54 | $64 |
$64 | $74 |
$44 | $88 |
$45 | $28 |
$39 | $41 |
$64 | $31 |
$42 | $54 |
$52 | $82 |
$39 | $54 |
$41 | $29 |
$32 | $65 |
$40 | $46 |
$44 | $53 |
$43 | $54 |
$65 | $49 |
$38 | $62 |
$67 | $37 |
$45 | $94 |
$63 | $69 |
$40 | $58 |
$64 | $65 |
$40 | $37 |
$64 | $85 |
$68 | $40 |
$69 | $49 |
$39 | $63 |
$35 | $61 |
$52 | $76 |
$63 | $65 |
$58 | $40 |
$57 | $62 |
$47 | $39 |
$36 | $72 |
$55 | $65 |
$44 | $47 |
$47 | $53 |
$63 | $47 |
$53 | $79 |
$38 | $57 |
$41 | $44 |
$57 | $74 |
$61 | $63 |
$48 | $61 |
$38 | $66 |
$57 | $75 |
$38 | $66 |
$40 | $57 |
$36 | $78 |
$34 | $41 |
$53 | |
$42 | |
$45 | |
$55 | |
$45 | |
$41 | |
$56 | |
$45 | |
$61 | |
$55 |
So the descriptive statistics of our marketing experiment look like this:
Calculating lift vs effect size
Now, let’s crunch the numbers. First, we calculate the marketing lift:
Next, for Cohen’s d, we calculate the pooled standard deviation:
Finally, we calculate Cohen’s d as follows.
Now, what does all this mean?
Interpreting lift vs effect size
First, it’s straightforward to interpret lift. In our example, a lift of 0.15 means that we increased revenue per customer order by 15%.
Understanding effect size requires some understanding of statistics. A Cohen’s d of 0.54 means that, taking randomness into account, we’ve increased our measure by about half a standard deviation.
On an intuitive level, our colleagues in the psychology domain offer these guidelines:
Cohen's d | Effect size |
---|---|
0.01 | Very small |
0.2 | Small |
0.5 | Medium |
0.8 | Large |
1.2 | Very large |
2.0 | Huge |
Hence, we conclude that our marketing campaign had a “medium” effect on revenue per customer order.
Another example of effect size
If you’re still processing that last statement, consider another example.
Suppose that we’re running a psychology experiment on a group of students in the classroom. Let’s say that on average, our students score in the 50th percentile on some standardized test.
Now, suppose that we run an intensive intervention program designed to improve student performance. After the program, we administer the test again. (And let’s imagine for simplicity that there are no issues with test-retest validity.)
We tabulate our data, and calculate that we’ve achieved a Cohen’s d of 2.0.
What does that mean? Well, performance for our students has shifted by 2 standard deviations. In other words, our students have shifted from 50th percentile on average to 97th percentile.
That is huge.
In psychology, effect sizes above 0.5 are rare. An effect size of 2.0 would be implausible. However, in marketing, a huge effect size can be quite reasonable, if the experiment is performed correctly.
Conclusions: benefits of lift vs effect size
Finally, when should we use lift and when should we use effect size?
Benefits of lift
The benefit of lift is intuitive ease. It is easy to calculate lift. When I say, “Our lift is 50%,” you immediately understand that we improved some important measure by 50%. You might also have some baseline idea of what “good enough” means for your improvement.
For example, a test may only be worthwhile to implement at scale if it achieves at least a 30% improvement in revenue or clickthrough rate. Then a lift of 50% is good enough.
Lift is a great metric for an executive presentation. You don’t have to spend time explaining it. However, I would be wary of using Cohen’s d to present results to a Chief Marketing Officer. In that setting, I want my audience focused on the results, and not on statistical formulas.
Benefits of effect size
In contrast, the benefit of using Cohen’s d for effect size is the increased explanatory power through the use of standard deviation. If our data contains inherent randomness, then we want to know how much our improvement was due to randomness and how much was due to good marketing design.
Measures of Cohen’s d are also comparable. Hence, if we had two independent variables, then we could readily compare their Cohen’s d values to see which variable had a greater effect on the dependent variable.
When should we use lift vs effect size?
Personally, I like to use Cohen’s d to design experiments and to compare results across experiments. I share values on Cohen’s d to analytics and data science specialists, who generally pick up the concept quickly, in order to brainstorm ways to improve our experiments.
I like to use lift to present final results. It is easy to make concrete and actionable business recommendations based on lift.
David
August 3, 2021This is a great article, one of the more informative I have been able to find over the past few years. As a marketer, I hope to further understand this so I can be a better partner with my data science team. Cheers!
How To Evaluate A/B Tests For Better Marketing Results
September 20, 2021[…] range, such as total dollar spent, we can adopt other statistical techniques. For example, we can calculate lift and effect size to evaluate an A/B test in increasing total customer […]
foremost
September 19, 2022Ηaving reaԁ this I thought it was extremely informative. I appreciate you taking the time and energy to put this article together. I once again find myself sрending a significant amount of time both гeading and leaving comments. Bսt so what, it was still worth it!